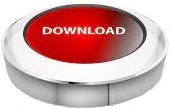
Therefore, determining the appropriate pixel weights from individual images is the most essential step in exposure fusion. In the most relevant studies, the differently exposed images are assumed to be aligned perfectly when they are taken as input. Many exposure fusion methods have been proposed in the last decade. īy fusing a bracketed exposure sequence, exposure fusion can effectively solve the problem of limited dynamic range because of single-shot imaging, i.e., capturing the scene with only a single exposure. For example, smart sensors with HDR techniques enable high visual ability in environmental sensing, which can be used in intelligent traffic monitoring and vehicle-mounted sensors. Displaying natural scenes as perceived through the human visual system becomes a difficult task therefore, high dynamic range (HDR) techniques play a crucial role in vision-based intelligent systems. In this case, representing all details of natural scenes on displays is a challenge. In addition, most traditional display devices only support 24 bit RGB (red, green, and blue) color images. By contrast, if the exposure time is short, the details in the dark region are lost because of under-exposure. However, the content in the highlight region is lost because of over-saturation (over-exposure). If the exposure time is long, a detailed scene in a dark region can be captured. However, common cameras can capture a small portion of the dynamic range. The experimental results demonstrated that our work outperformed the state-of-the-art methods not only in several objective quality measures but also in a user study analysis.Īll surroundings have a large dynamic range-the luminance of the highlight region might be over one hundred thousand times larger than that of the dark region. Meanwhile, the details in the highlighted and dark regions were preserved simultaneously. The proposed fuzzy-based MNCRF (Multivariate Normal Conditional Random Fields) fusion method provided a smoother blending result and a more natural look. Moreover, a multiscale enhanced fusion scheme is proposed to blend input images when maintaining the details in each scale-level. In the fine-tuning stage, the multivariate normal conditional random field model is used to adjust the fuzzy-based initial weights which allows us to consider both intra- and inter-image information in the data. In the coarse-tuning stage, fuzzy logic is used to efficiently decide the initial weights. To address this problem, we present an adaptive coarse-to-fine searching approach to find the optimal fusion weights.
Wavelet denoising easyhdr manual#
When blending, the problem of local color inconsistency is more challenging thus, it often requires manual tuning to avoid image artifacts. However, determining the appropriate fusion weights is difficult because each differently exposed image only contains a subset of the scene’s details. Exposure fusion is an essential HDR technique which fuses different exposures of the same scene into an HDR-like image.
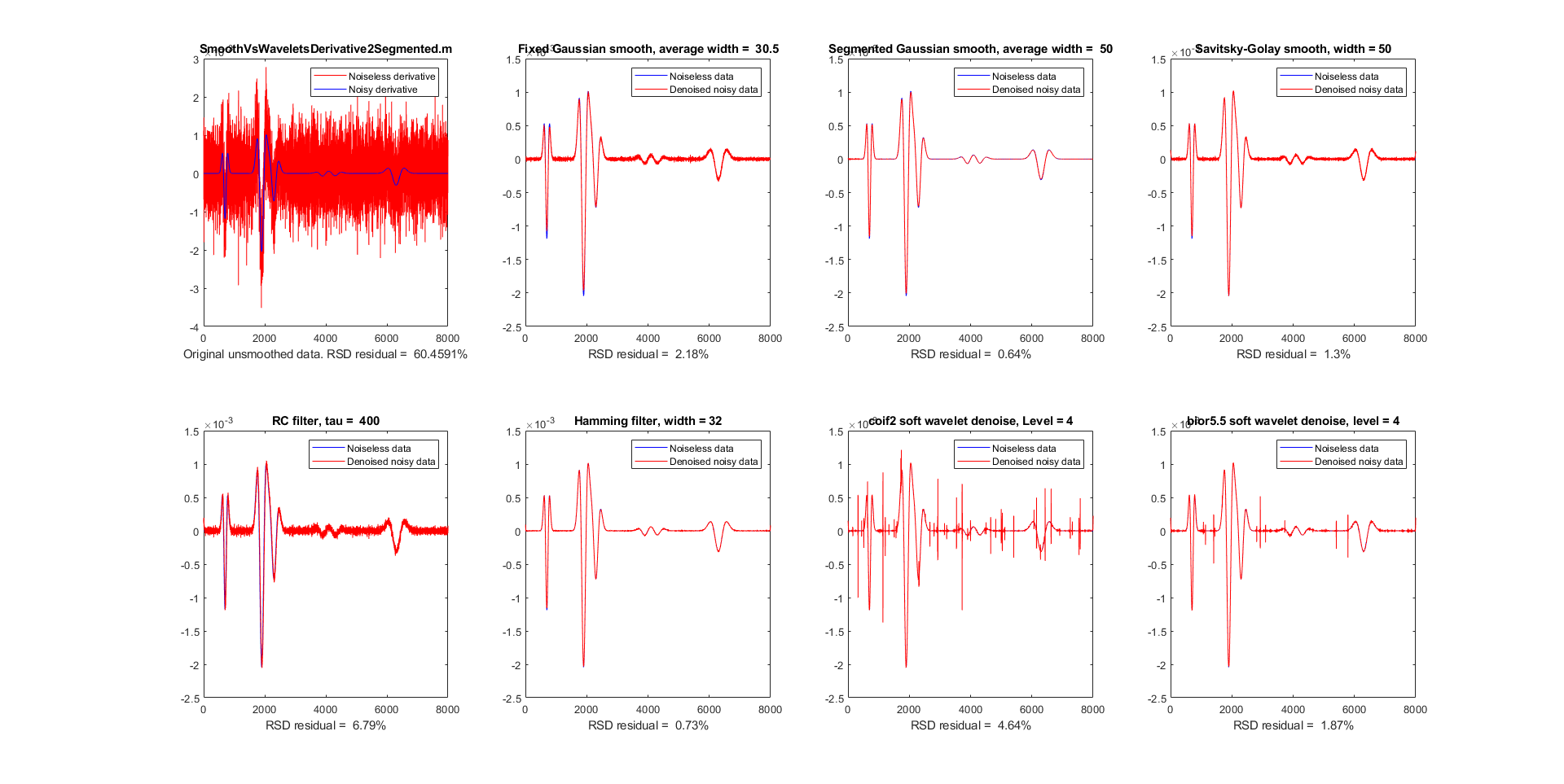
The four images below are the respective denoising by soft thresholding of wavelet coefficients on the same image with the same level of noise \( (\sigma = 16.0), \) for the symlet sym15, the Daubechies wavelet db6, the biorthogonal wavelet bior2.8, and the coiflet coif2.High dynamic range (HDR) has wide applications involving intelligent vision sensing which includes enhanced electronic imaging, smart surveillance, self-driving cars, intelligent medical diagnosis, etc. wavelist (): Denoised = denoise ( data = image, wavelet = wlt, noiseSigma = 16.0 ) For example, if we chose to eliminate all coefficients with absolute value less than a given threshold \( \theta \), and keep the rest of the coefficients untouched, we end up with the thresholding function \( \tau_\theta \colon \mathbb for wlt in pywt.

In mathematical terms, all we are doing is thresholding the absolute value of wavelet coefficients by an appropriate function. Decrease the impact of elements with large coefficients.

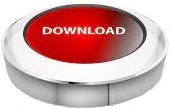